

Ideally, most of your clustering variables will be quantitative, although you may also include some binary variables. If most or all of your previous explanatory variables are categorical, you should identify some additional quantitative clustering variables from your data set. You can use the same variables that you have used in past weeks as clustering variables. Finally, you will get the opportunity to validate your cluster solution by examining differences between clusters on a variable not included in your cluster analysis. You will gain experience in interpreting cluster analysis results by using graphing methods to help you determine the number of clusters to interpret, and examining clustering variable means to evaluate the cluster profiles.
Machine learning tools for data analysis how to#
In this session, we will show you how to use k-means cluster analysis to identify clusters of observations in your data set. Clustering variables should be primarily quantitative variables, but binary variables may also be included. The goal of cluster analysis is to group, or cluster, observations into subsets based on their similarity of responses on multiple variables. The cross-validation method you apply is designed to eliminate the need to split your data when you have a limited number of observations.Ĭluster analysis is an unsupervised machine learning method that partitions the observations in a data set into a smaller set of clusters where each observation belongs to only one cluster. Note also that if you are working with a relatively small data set, you do not need to split your data into training and test data sets. The lasso regression analysis will help you determine which of your predictors are most important. Take some chances, and try some new variables. Remember that lasso regression is a machine learning method, so your choice of additional predictors does not necessarily need to depend on a research hypothesis or theory. Having a larger pool of predictors to test will maximize your experience with lasso regression analysis.

explanatory) variables to develop a larger pool of predictors. To test a lasso regression model, you will need to identify a quantitative response variable from your data set if you haven’t already done so, and choose a few additional quantitative and categorical predictor (i.e. You will also develop experience using k-fold cross validation to select the best fitting model and obtain a more accurate estimate of your model’s test error rate. In this session, you will apply and interpret a lasso regression analysis. Explanatory variables can be either quantitative, categorical or both. Variables with non-zero regression coefficients variables are most strongly associated with the response variable. Variables with a regression coefficient equal to zero after the shrinkage process are excluded from the model. The lasso does this by imposing a constraint on the model parameters that causes regression coefficients for some variables to shrink toward zero.
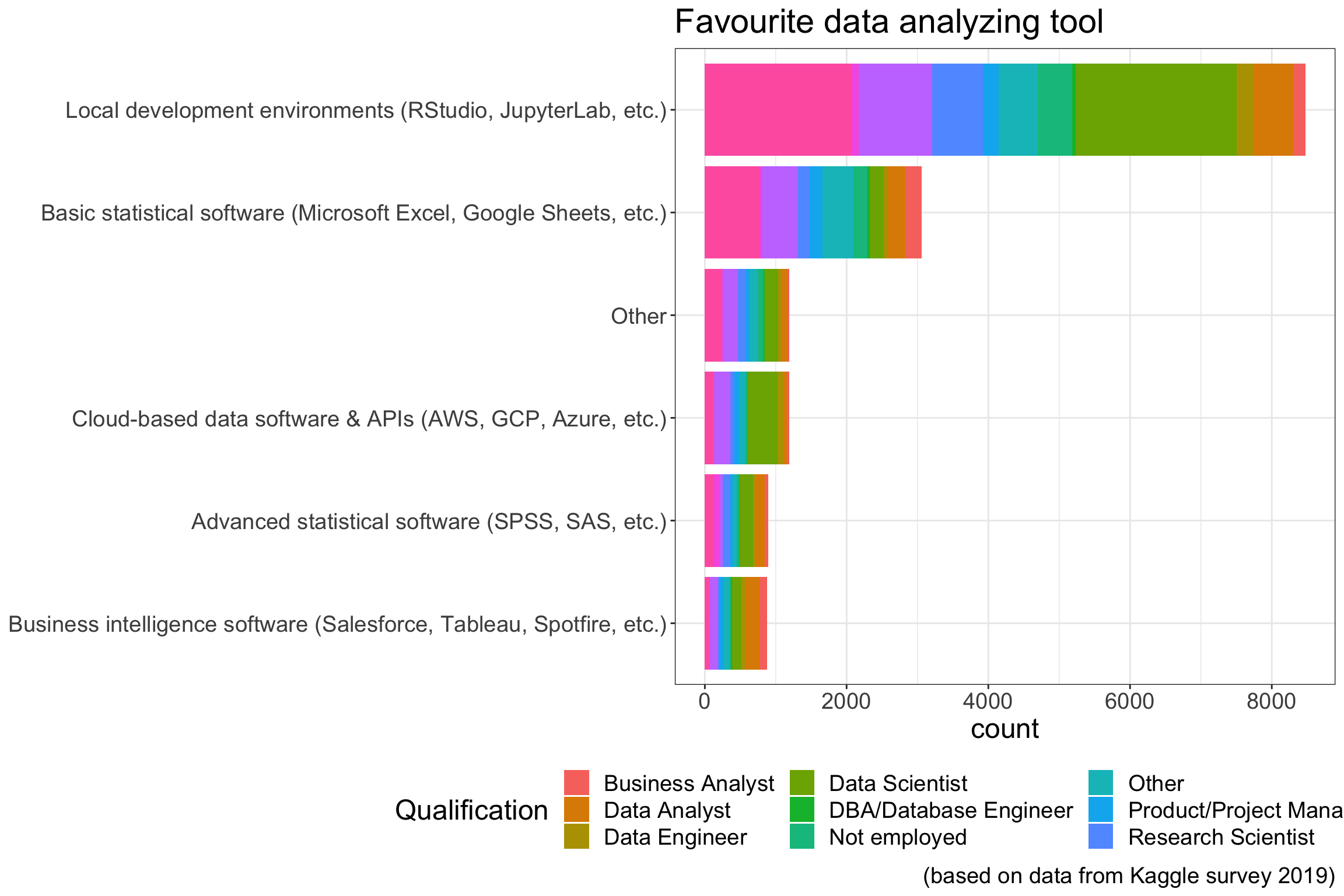
The goal of lasso regression is to obtain the subset of predictors that minimizes prediction error for a quantitative response variable. Lasso regression analysis is a shrinkage and variable selection method for linear regression models.
